As designers, even those of us who don’t work “in tech” are immersed in tech. Some of us are eager early adopters. Many live with a simmering FOMO when everyone else seems keyed in to industry sea changes we just don’t get.
And we’re all subject to the way the products we use to work (or work to build) are trying to cash in on the current craze.
With Web3 going down in flames, that current craze is AI (or machine learning models if you think it’s a bit premature to attribute intelligence to Google Bard).
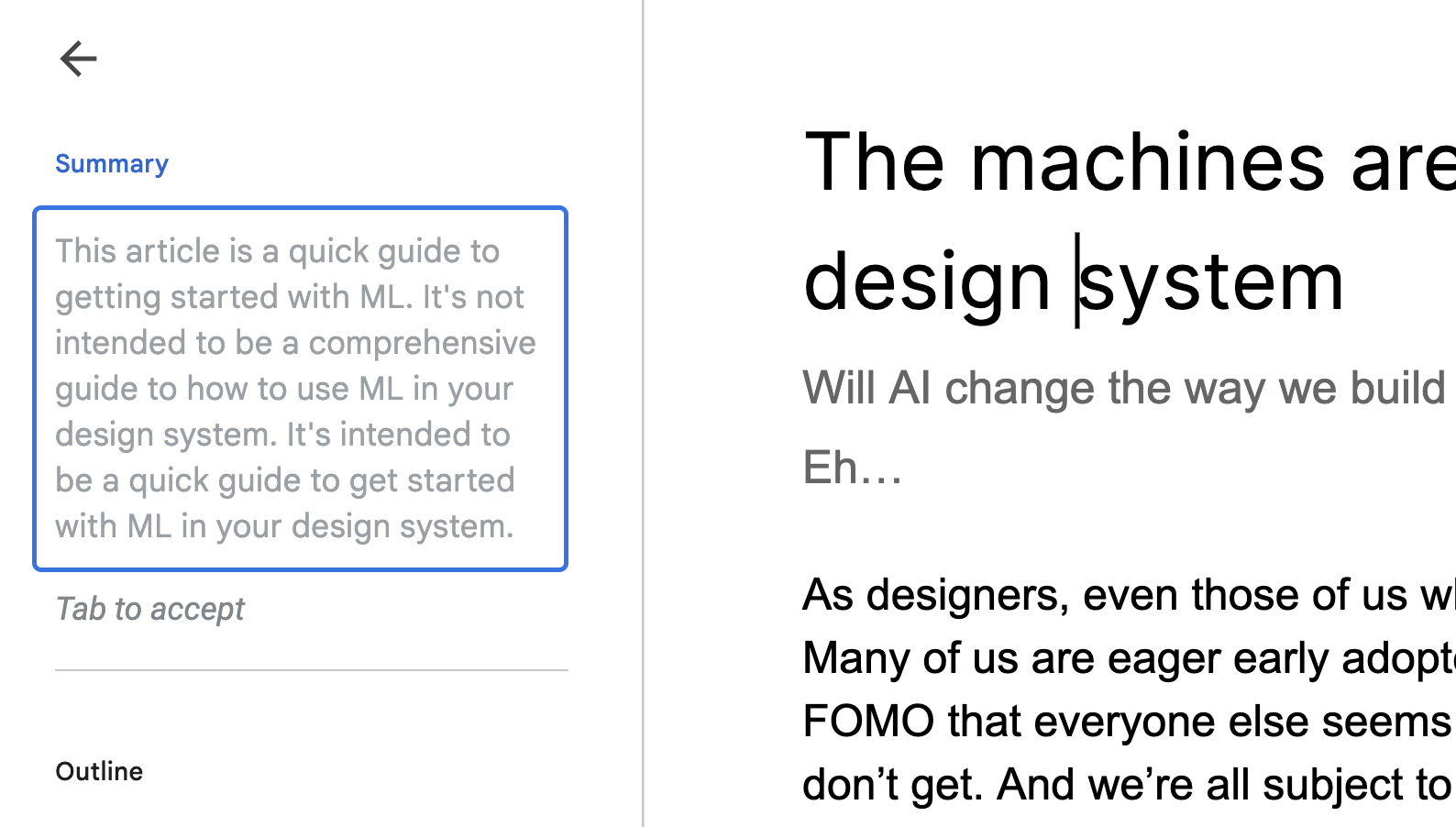
Every day, a new marketing email, Medium post, or VC who will leave Twitter when they’re cold in a body bag tells us that machine learning (ML, which they call AI because it sounds more expensive) is going to change the way we work. Doesn’t really matter what your job is. ML is going to read, write, code, and paint for us.
Naturally, the excitement around ML has found its way into the design systems community. There’s an apparent natural synergy between ML and design systems. Design systems practitioners are tantalized by the promise of even greater efficiency and scale. We wish a machine would write our docs for us.
We are all, every single one of us, huge fucking nerds.
Maybe it is inevitable that ML will change the way we build design systems. If not on its merits, then as part of a self-fulfilling hype cycle—where it does because enough people believe it will.
So you get feverish articles that tell you how to get ChatGPT to generate a color palette for you, create user personas, or write your API specs.
And look, I don’t begrudge anyone their excitement. I don’t think it’s bad to look at nascent technology and dream of what could be. That speculation may lead to truly interesting, beneficial uses of ML.
Where the danger lies for the design systems practice is confusing what could be in some shining future with what is in our messy today.
There is a wide chasm between the speculative future these articles depict and where ML can take us in its current form. If we’re not careful, design systems practitioners are going to Wile E. Coyote our way to the bottom of that chasm.
Yesenia Perez-Cruz writes, “The next wave of design systems will be AI-driven.” As a design systems pioneer, she identifies “Sameness over cohesion,” “Assembly over purpose,” and “Decisions divorced from where work happens,” as perennial challenges ML can help address.
She suggests a future in which a designer can prompt an ML trained on your design system with the purpose of a design and receive the appropriate pattern back. It’s a very cool idea to which many companies would like to sign you up for an Enterprise subscription.
Unfortunately, achieving cohesion without sameness, creating with purpose, and holistic decision making are things ML is uniquely mispositioned to address.
A machine can only give you an amalgamation of what’s been put into it, which it evaluates not against intent but plausibility.

It will spit out a pattern that matches the prompt, but it won’t consider how that same intent has been realized elsewhere in your product, upcoming shifts in company strategy, or patterns that other teams are working on right now that haven’t been fed into its training data.
ML is better suited to the types of tasks Brad Frost describes. Tell it what to build and “splat goes the AI.”
By all means ChatGPT can have fun configuring Figma auto layout and variant settings for me once a design solution is ready to be put into production; but what happens when someone on your team prompts a tool that can only splat out what it’s been told already to solve a novel problem like it’s an Intelligent designer?
I think they’ll get something that looks like a system but has no articulated reason underneath it. We’ve seen plenty of those created by people. They’ll probably be in its training set.
The designers who don’t know how to use your design system now will be no more capable of evaluating the output quality of an ML trained on those systems then.
In the (gold) rush to bring machine learning into our design systems, we can’t forget that design systems are for people.
Before an AI can build a UI, a human designer on your team needs to be able to tell it what to build. Before an AI can suggest patterns that map to specific design intents, the designer needs to know how to frame the intent of their design.
How you frame success matters.
If you’ve worked with more than a handful of product managers you know how a feature intended to solve a client problem changes when that same feature is optimized to move a metric.
An ML will build what you tell it but not challenge why you’re building it. It has no ethics that haven’t been set as an explicit-if-half-assed guardrail, and it’s even less aware of its biases than we are.
Why are our dreams for AI built around products that help people think less? Why not faster, clearer, bigger?
Like a chatbot parroting the racism of the people with whom it chats, thoughtless application of ML to design systems can amplify the things people dislike most about them. The sameness, the lack of designer agency, a focus on production over problem solving.
There’s a reason the articles touting ML for design systems focus on efficiency and scale—and not quality and efficacy. If we accept that limited framing of success for our practice, we risk doing serious damage to our field, our products, and the people who use them.
We have too much confidence in our ability to make technology better, and not enough respect for its ability to make our lives worse.
Maybe it is inevitable that ML will change the way we build design systems. If not on its merits, then as part of a self-fulfilling hype cycle—where it does because enough people believe it will.
And maybe one day we will cross that chasm between what ML is capable of today and the automated space utopia its proponents envision.
Right now, though, we have too much confidence in our ability to make technology better, and not enough respect for its ability to make our lives worse.
I know we won’t escape "How AI will change Design Systems" articles; but let’s try to keep our genuine intelligence about us in the meantime.